On the second day of the RIMS virtual event TechRisk/RiskTech, CornerstoneAI founder and president Chantal Sathi and advisor Eric Barberio discussed the potential uses for artificial intelligence-based technologies and how risk managers can avoid the potential inherent biases in AI.
Explaining the current state of AI and machine learning, Sathi noted that this is “emerging technology and is here to stay,” making it even more imperative to understand and account for the associated risks. The algorithms that make up these technologies feed off data sets, Sathi explained, and these data sets can contain inherent bias in how they are collected and used. While it is a misconception that all algorithms have or can produce bias, the fundamental challenge is determining whether the AI and machine learning systems that a risk manager’s company uses do contain bias.
The risks of not rooting out bias in your company’s technology include:
- Loss of trust: If or when it is revealed that the company’s products and services are based on biased technology or data, customers and others will lose faith in the company.
- Punitive damage: Countries around the world have implemented or are in the process of implementing regulations governing AI, attempting to ensure human control of such technologies. These regulations (such as GDPR in the European Union) can include punitive damages for violations.
- Social harm: The widespread use of AI and machine learning includes applications in legal sentencing, medical decisions, job applications and other business functions that have major impact on people’s lives and society at large.
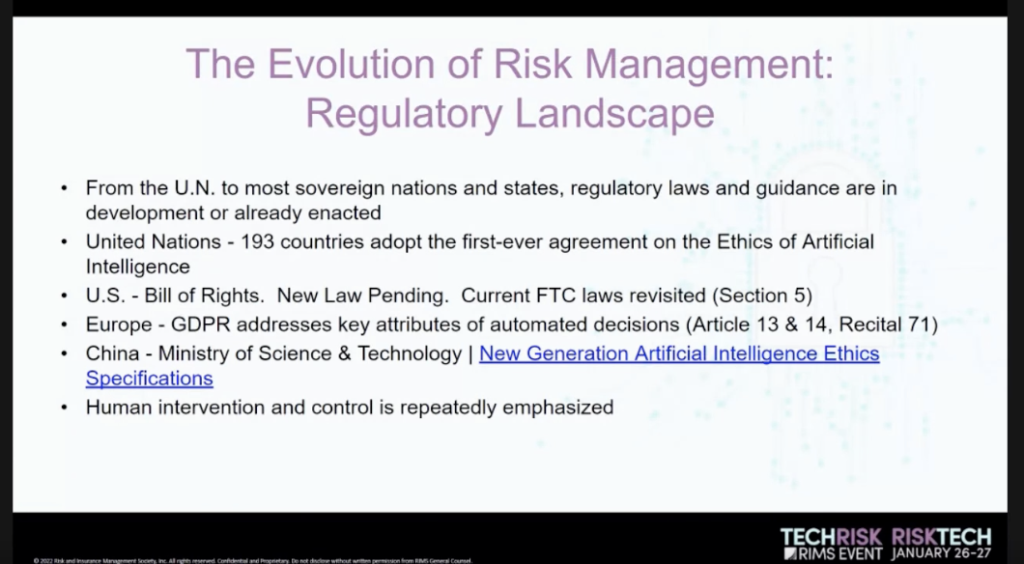
Sathi and Barberio outlined five steps to assess these technologies for fairness and address bias:
- Clearly and specifically defining the scope of what the product is supposed to do.
- Interpreting and pre-processing the data, which involves gathering and cleaning the data to determine if it adequately represents the full scope of ethnic backgrounds and other demographics.
- Most importantly, the company should employ a bias detection framework. This can include a data audit tool to determine whether any output demonstrates unjustified differential bias.
- Validating the results the product produces using correlation open source toolkits, such as IBM AI Fairness 360 or MS Fairlearn.
- Producing a final assessment report.
Following these steps, risk professionals can help ensure their companies use AI and machine learning without perpetuating its inherent bias.
The session “Emerging Risk AI Bias” and others from RIMS TechRisk/RiskTech will be available on-demand for the next 60 days, and you can access the virtual event here.